TAS: Ternarized Neural Architecture Search for Resource-Constrained Edge Devices
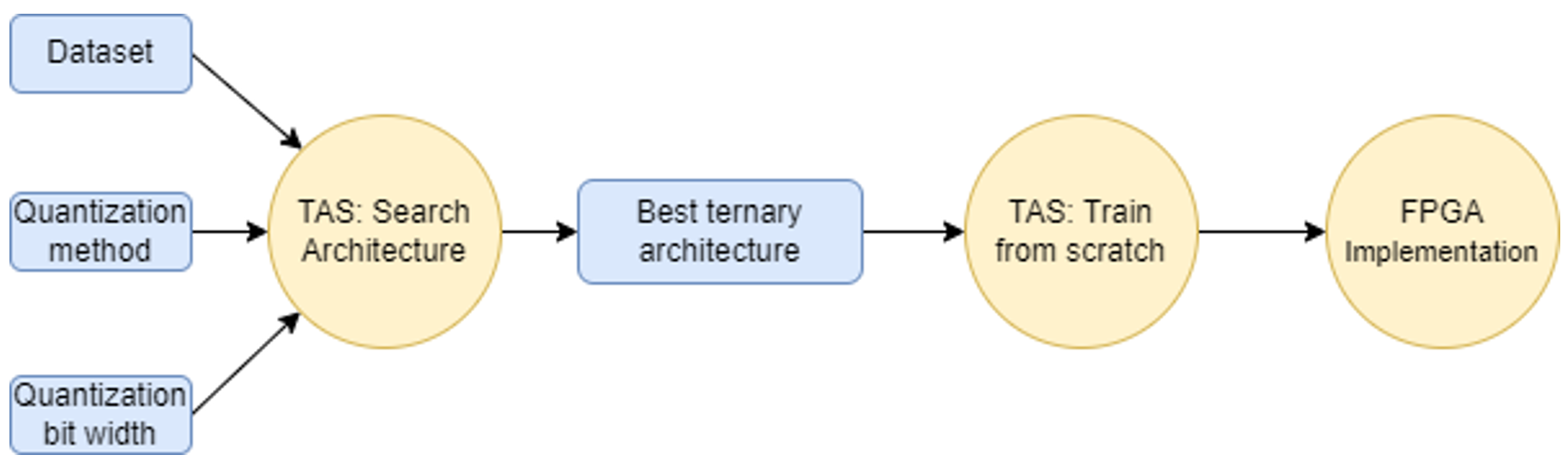
1. Search Architecture: This stage will automatically search for the best architecture for the specific dataset, quantization method and quantization bit width.
2. Train from scratch: To obtain the final performance, one must train the best architecture from scratch.
3. FPGA Implementation: To evaluate the TAS performance on real hardware, we deploy the best architecture on FPGA using the DeepHLS framework [3].
References:
[1] Loni, Mohammad, et al. “Faststereonet: A fast neural architecture search for improving the inference of disparity estimation on resource-limited platforms.” IEEE Transactions on Systems, Man, and Cybernetics: Systems (2021).
[2] Loni, Mohammad, et al. “TAS: Ternarized Neural Architecture Search for Resource-Constrained Edge Devices.” 2022 Design, Automation & Test in Europe Conference & Exhibition (DATE). IEEE, 2022.
[3] Riazati, Mohammad, et al. “DeepHLS: A complete toolchain for automatic synthesis of deep neural networks to FPGA.” 2020 27th IEEE International Conference on Electronics, Circuits and Systems (ICECS). IEEE, 2020.